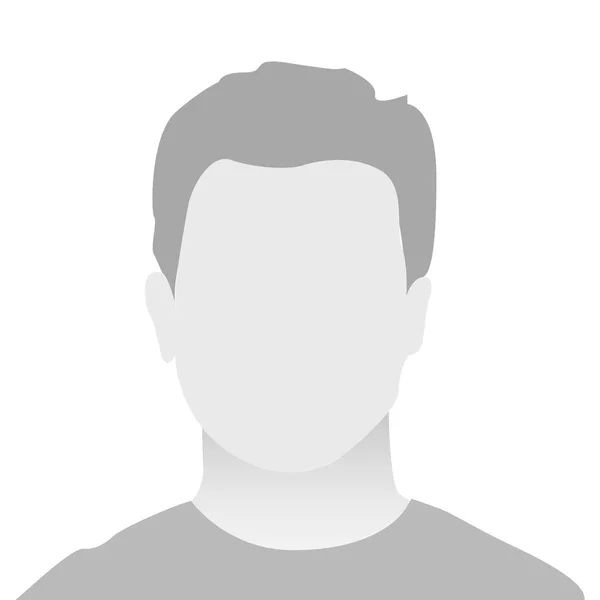
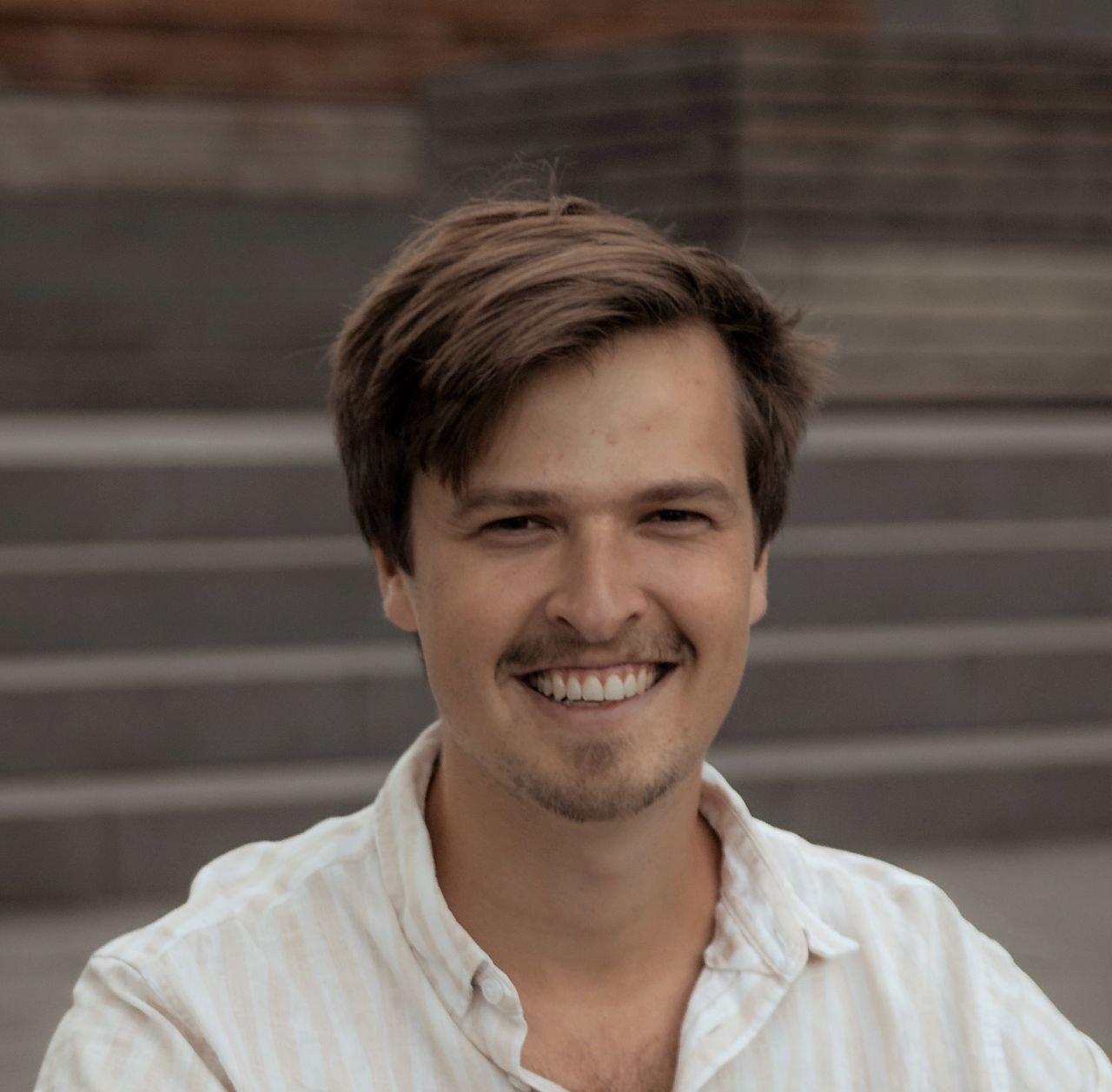



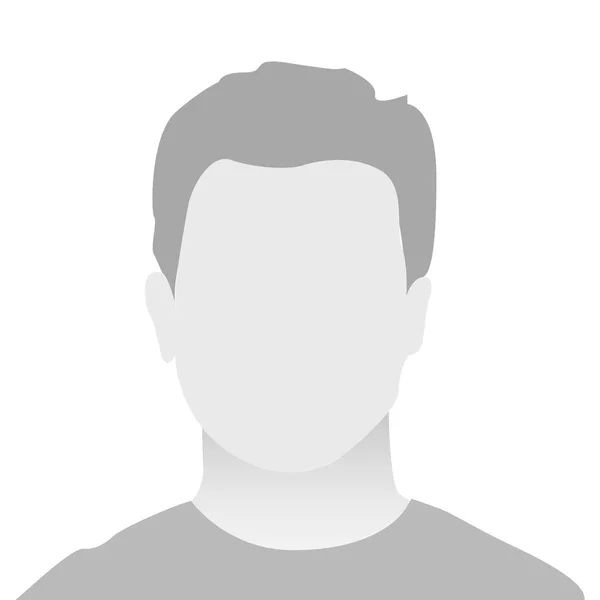

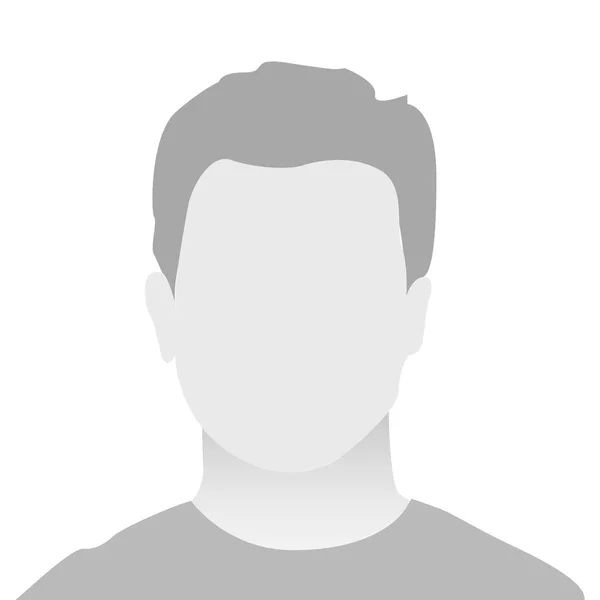
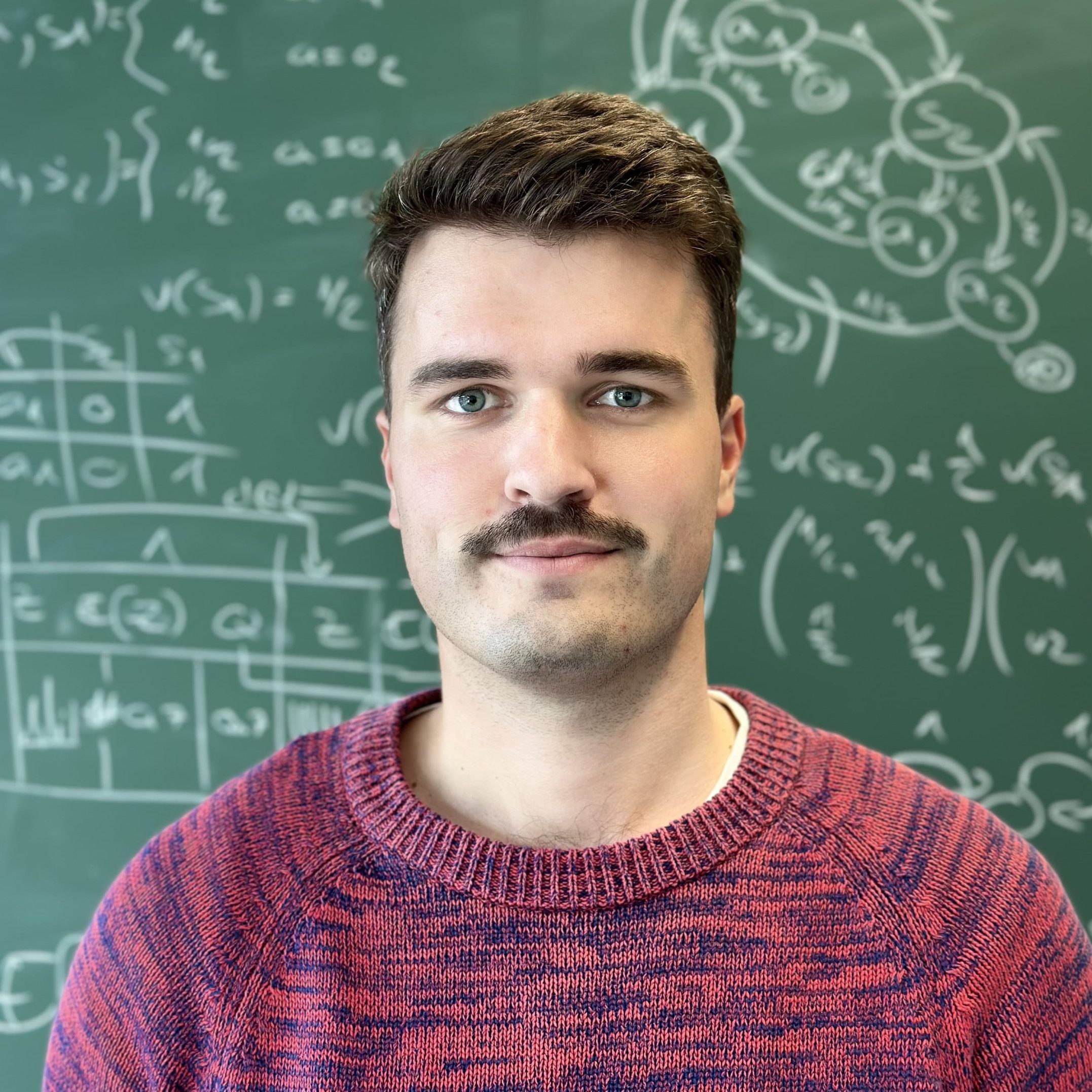
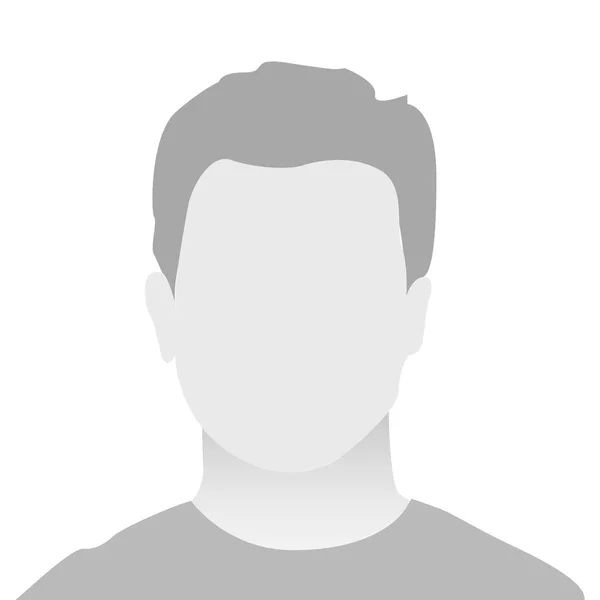

As a physicist, I seek to gain a mechanistic understanding of the functionality of the human brain and the resulting behavior. Depending on the resolution of the examined data, behavior is to be understood as an emergent phenomenon of the interactions of individual neurons, populations of neurons or whole brain regions. With the help of generative neural networks, I reconstruct empirical neuronal activity and use the resulting models as surrogates for the underlying dynamical system. They can then be used for in silico experiments to simulate the response of the brain to artificial conditions and thus draw conclusions about the physiology.
Besides that, I am interested in the development of deep learning models for the prediction of time series and the reconstruction of dynamical systems in general.
I spend my free time doing sports (running, hip hop, cycling) or let my thoughts run free while hiking. You can reach me at niklas.emonds@iwr.uni-heidelberg.de.
My recent research has focused on developing machine learning algorithms for extracting dynamical systems models from empirical time series data. One of my main motivations was to make these approaches work in real world clinical settings, including multi-subject data, very short, noisy, and non-Gaussian time series. A novel research direction I want to pursue is the intersection of creativity and generative AI.
Outside of research, I enjoy many things art-related (making music with my jazz trio triolog, literature, cinema), as well as cooking, playing chess and traveling.
I studied psychology and mathematics. My research interprets psychological processes as dynamical systems that can be analyzed and controlled. I develop and apply recurrent neural networks, control theoretic, and reinforcement learning methods to time series data of human affect and behaviour. My goal is to optimize interventions to improve mental well-being, and to gain a better understanding of these inherently nonlinear processes.
I am a Postdoctoral fellow at the AI Health Innovation Cluster, working with the University of Heidelberg and the ZI Mannheim to develop computational models of social interactions for adaptive gamified treatment approaches. I hold an MSc in Cognitive and Decision Sciences, an MRes in Computer Science (Machine Learning) from University College London, and a PhD in Experimental Psychology, specializing in experimental and computational approaches to strategic social interaction. My research focuses on social learning in mental health disorders, particularly exploring how cognitive interventions from leading psychotherapies can be integrated with computational behavior models to address social learning dysfunction.
Outside office hours, you’ll probably find me hiking around the hills in Heidelberg.
I’m a Data and Computer Science student at the University of Heidelberg, with a strong background in software development and machine learning. I’ve worked on web development, iOS apps, computer games, and full-stack projects. My current research adventures include diving deep into topics like Large Language Models and Retrieval-Augmented Generation—yep, the cool AI stuff!
I’ve studied in Germany, Italy, and India, and I’m always excited to take on new challenges and build scalable solutions.
When I’m not geeking out over code, you’ll find me in the kitchen whipping up some Indian cuisine (seriously, hit me up if you’re hungry). I also love jamming on the piano or violin, swimming, and occasionally crushing it at table tennis!
My work is driven by a deep curiosity to uncover the underlying mechanisms of human behavior, ranging from individual cognitive functions to complex social dynamics. Through machine learning and computational models, we aim to bridge the gap between human cognition and societal interactions, advancing both scientific understanding and practical applications.
I studied mathematics at the University of Mannheim, where I was first introduced to both reinforcement learning and generative time series modelling, sparking my interest in their algorithmic foundations and applications to computational neuroscience.
My current focus is on using reinforcement learning models to better understand the psychological and neuroscientific processes behind human decision-making, particularly in social interactions and psychiatric disorders.
I’m also exploring how reinforcement learning concepts can be integrated with dynamical system reconstruction, especially in the presence of chaos and randomness.
Outside of academics, I enjoy playing and coaching Ultimate Frisbee on an international level.